Article: Artificial intelligence at DB
Fewer delays, more efficient maintenance and better customer service thanks to AI. Artificial intelligence (AI) is no longer a distant vision of the future at Deutsche Bahn. In various Group-wide projects, processes relating to data and AI are already having an impact. They are not only making digitalization tangible but also represent a powerful tool to make rail even more appealing.
The use of AI begins with the planning of materials, encompasses the smart control of S-Bahn services and extends to the digitalization of maintenance. In just one of its applications, AI helps dispatchers manage S-Bahn services more efficiently during disruptions. After a successful pilot test of the technology in the Stuttgart S-Bahn system, DB is also introducing an internally developed AI-based tool in the Rhine-Main and Munich S-Bahn systems. This tool generates recommendations for DB staff that facilitate a more proactive approach to managing irregularities in S-Bahn operations and to thus reduce delays to a minimum. That, in turn, helps eliminate the build-up of waiting times and traffic jams on busy routes. In Stuttgart, the tool enables DB to compensate for delays of up to eight minutes. DB also wants to use the resource it has developed to create capacity for more trains.
The deployment of AI has also proven increasingly useful in predictive maintenance. DB's goal here is the wholesale implementation of condition-based maintenance for trains. This type of servicing reduces workloads for employees and cuts the times for physically elaborate tasks such as ICE roof inspections from several hours to just a few minutes. In diagnostics, AI technologies identify specific maintenance requirements by automatically evaluating camera images and sensor data.
Other projects in maintenance are aimed at reliably predicting the optimum times for servicing vehicle parts. Here, DB is currently developing an AI-based tool that uses data-driven forecasting to enable S-Bahn maintenance planners to schedule wheelset replacement and conditioning more effectively, thus saving on resources and costs and helping to increase vehicle availability.
DB also uses AI processes to improve the travel information it provides on the Deutsche Bahn website, DB Navigator, at the stations and on other customer channels. Information on expected train arrival and departure times, for example, are vital for travelers, and DB is using AI-driven forecasting based on historical and real-time data to better predict these events. DB is also using AI to develop virtual assistants for customer service. In addition to text-based chatbots, as used on bahn.de for example, DB has also created a smart interactive voice response system called SEMMI and tested it in customer service.
AI in S-Bahn systems
For our S-Bahn regional trains, DB uses AI in the operations centers for more efficient control of S-Bahn traffic during disruptions. Here dispatchers monitor the movement of trains and must respond quickly to any irregularity. For example, if departure is delayed due to the slow boarding of a school class, and the train reaches a single-track section at the same time as another train, the AI system calculates in a fraction of a second which train should pass through the section first. Dispatchers can fast-forward to a possible future scenario just as if it were a video clip, and see how recommendations will affect traffic before making a decision.
Furthermore, AI continuously analyzes live operations to simulate potential developments in the rail traffic situation and report possible conflicts at an early stage. In the event of irregularities, the system enables dispatchers to take preventive action that was not possible in the past and thus manage S-Bahn traffic so that delays are kept to a minimum.
A better flow of traffic is the result: since trains no longer have to reduce their speed or wait as frequently when another train blocks a line section, the waiting times and traffic jams on busy routes can be avoided. In Stuttgart, DB can compensate for delays of up to eight minutes. DB also wants to use its in-house development to create capacity for more trains so it can make better use of existing infrastructure. In theory, for example, artificial intelligence could be used to run 17 more trains per day in each direction on Stuttgart's core route.
SEMMI
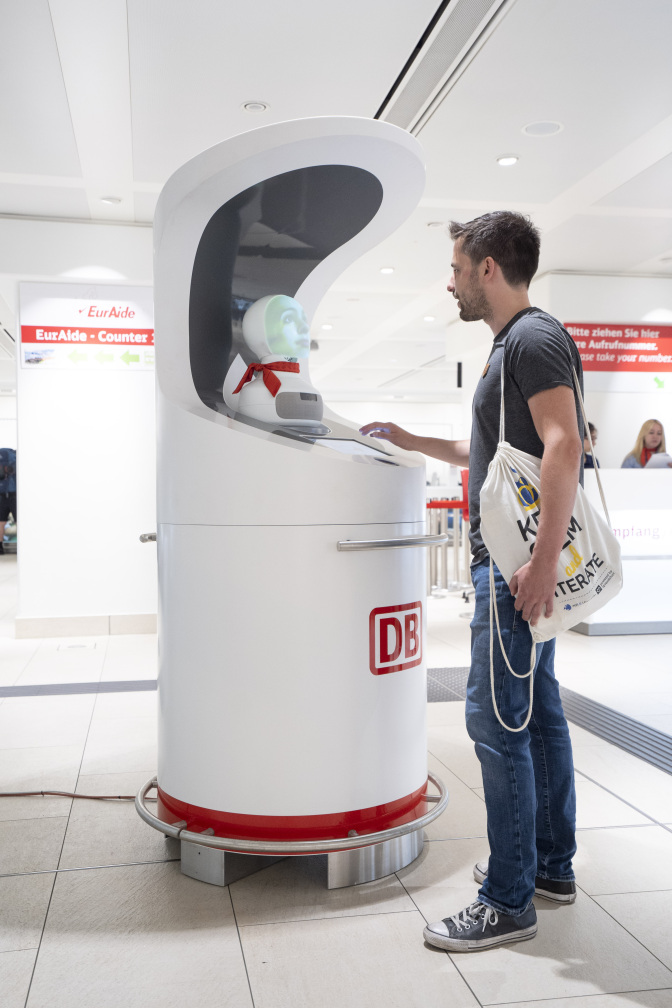
SEMMI (short for Socio-Emphatic Human-Machine Interaction) is an AI-based interactive voice response system that can be used in a variety of ways: as a digital avatar, a voice bot on a telephone hotline or even as a robot in customer service. Taking the form of a female robot, SEMMI supported service staff in tests held at Berlin Central Station's travel center and Frankfurt Airport in 2019. SEMMI first appeared in the form of a digital avatar in 2021 when DB tested the system at the Stephansplatz subway station in Hamburg together with HOCHBAHN, the city’s elevated railroad company, and at Newcastle station in the UK together with the British rail company LNER.
SEMMI received its knowledge from DB developers, who geared up the robot head to answer traveler questions. DB is also working together with TH Köln University of Applied Sciences on the development of a socio-empathic conversation system called "conversational AI." Today's voice-based systems can only understand commands and simple spoken queries. Communication on an equal footing, however, can only be achieved with conversational AI, which is able to express appreciation, for example. Here, AI-researchers want to accomplish more than just technological advancement; they want to explore human-machine interaction in greater detail. One issue they face is the question of how machines must present themselves so that users feel they have received good advice. Examples are gestures and facial expressions as well as cultural customs. More information is available here.
Artificial intelligence in maintenance
AI is currently being tested and applied to maintenance tasks such as visual diagnostics and condition-based maintenance across Deutsche Bahn. The goal here is to increase maintenance capacities by exploiting the interaction of AI and automation. For example, cameras at DB Long Distance, DB Regio and DB Cargo will be used to evaluate vehicles with the help of automated image analysis, a process that harnesses AI-based digital image processing to identify train damage in just a few minutes.
The images are taken while the train passes under a camera bridge. Maintenance employees then receive the AI’s results, which include notes on any vehicles in need of maintenance. The end result is a much more effective maintenance staff who no longer have to physically walk around trains looking for damage but are instead alerted to any piece of equipment performing outside normal parameters, i.e., the asset’s conditional baseline. Furthermore, photos can be used as a type of "remote monitoring" to reduce time-consuming physical tasks such as climbing onto a train car roof.
The company is also testing a forecasting tool for regional trains to vastly improve early material requirements planning for wheelset maintenance. Ultimately, it is clients who benefit. Since DB capacities are channeled less into troubleshooting and more into repairs, passengers get to enjoy higher quality – i.e. better maintained – vehicles.
Peak spotting
AI is also being deployed in the form of a cutting-edge data visualization tool called “Peak Spotting," which is used to improve passenger management by distributing demand as optimally as possible among trains. Peak spotting uses a suite of visual data-informed tools and visualizations to identify ("spot") looming peaks in capacity utilization on long-distance trains and at stations and thus enable timely interventions. Traffic managers can then deploy high-capacity passenger trains primarily to areas of spiking demand and passengers can receive timely information about the expected high occupancy rates. Behind Peak Spotting is powerful predictive software based on machine-learning algorithms. On-going development is improving the reliability of the tool's capacity predictions and thus improving the information we can provide to our clients. In this form, AI offers immediate payoffs in improved capacity management.
The smart way to forecast delays
For travelers, it's of utmost importance to know when a train is expected to depart or arrive. It's even more important for the information to be accurate and available well ahead of time. Deutsche Bahn's passenger information forecasting improves the quality of the passenger information that we publish on our customer channels, offering greater reliability and convenience for travelers.
The tool leverages big data technologies and artificial intelligence methods to calculate the arrival and departure times for train connections. It draws on a variety of real-time and historical data sources such as customer timetables, operational timetables, and actual data from network signaling systems. The historical data is fed into machine-learning algorithms to "train" forecasting models that identify delay patterns. The models are then combined with real-time data to predict current train movements.
The primary advantage of passenger information forecasting is that delays and changes in delays can be detected at an early stage, enabling DB to provide passengers with timely, up-to-date information. In addition, this tool also takes into account interactions between multiple trains, since the delay of one train is often caused by another. This allows accurate forecasts to be made for common transport situations such as train sequencing conflicts on the line.
Passenger information forecasting is thus comprehensive, keeping a watchful eye on the whole of rail transport. The result is a reliable real-time travel assistance system that has been proving its value to customers since 2018 and that is currently used in the train dispatch AI project.